In an era of digital transformation, it’s more important than ever for companies of any size to have proper data management. Understanding historical data and the information that is pouring in real-time can help organizations get the most data value to make better business decisions based on analytics and insights.
Also Read: The Importance of Data Science for Business
However, data quality is crucial to make sure that the right information is being used to form those analytics and insights. Here is what you need to know when it comes to getting the highest-quality data.
Understanding Data Quality

Data quality refers to when data fits the purpose it’s intended for. It’s considered high quality when it accurately represents real-world constructs. Bad quality data results in bad information, which is then spread throughout the hierarchy of an organization, leading to bad business decisions.
Data accuracy is crucial to making the right next steps, and that’s why it’s important to understand data quality dimensions. There are numerous ways to ensure that data is not duplicated, from the entry point to the deduplication techniques of data already stored in databases.
Also Read: How to migrate your ERP data
There are six primary dimensions of data quality: comprehensiveness, consistency, accuracy, format, timeframe, and integrity. Comprehensiveness looks into the essential fields that need to be filled in a data set. Consistency ensures iterations of that report are the same across all access points.
Accuracy deals with the correctness of the specifics of that data. Format refers to a quality assessment of this information in one specified design. Timeframe reflects on the real-time access and delivery of these data sets, and integrity is the safety and compliance regulations upheld for proper data governance.
Data Assessments
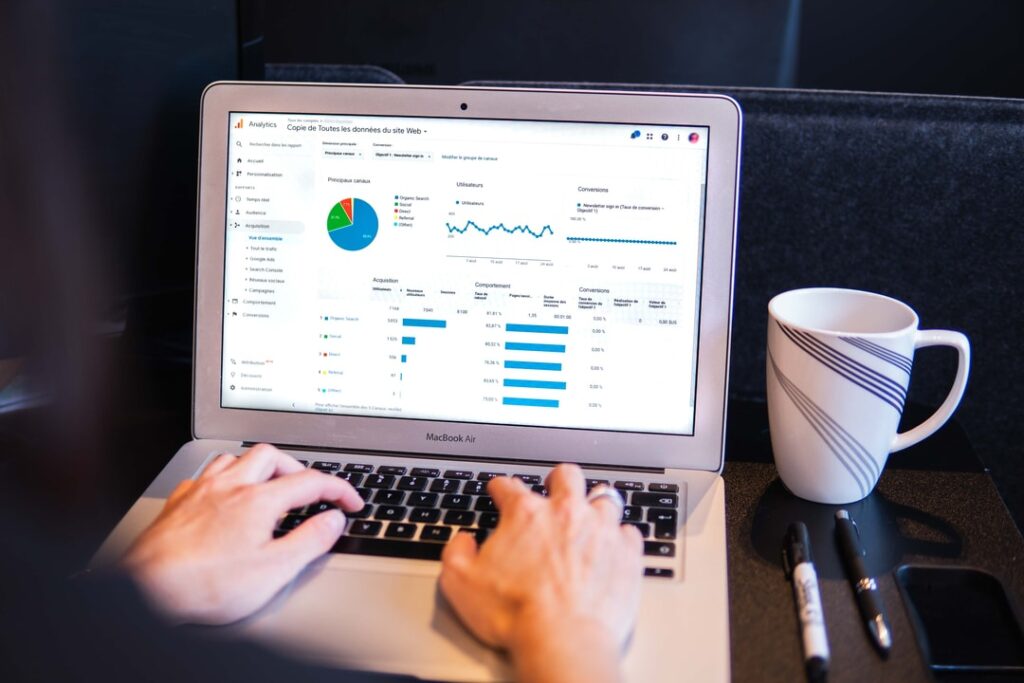
Given that organizations stand to lose considerably if business processes are based on bad quality data, it becomes crucial to understand how data quality is being assessed. This task includes setting up metrics and processes needed to work on making data rank highly for both objective and subjective assessments. With subjective assessments, organizations measure how parties perceive the quality of data.
If any decision is made based on the data they receive that is later revealed to have inaccurate or invalid information, then the decision is impacted. This has to be taken into consideration when looking to find loopholes in the quality of data.
Also Read: Learn About Technology That Can Keep Your Company’s Data Secure
Objective data quality assessments refer to performance within a specific task or from a viewpoint that it is a metrics-based data set that can be used independently. To set these metrics, organizations can work on principles to develop key performance indicators (KPIs) that match their specific needs. This can be measured through simple ratios, minimums or maximums, or weighted averages.
A simple ratio refers to the total number of desired outcomes within data sets within total possible outcomes to mete out completeness. Minimums and maximums handle multiple data quality variables while a weighted average is used to understand the value of the equation.
Benefits of Data Quality
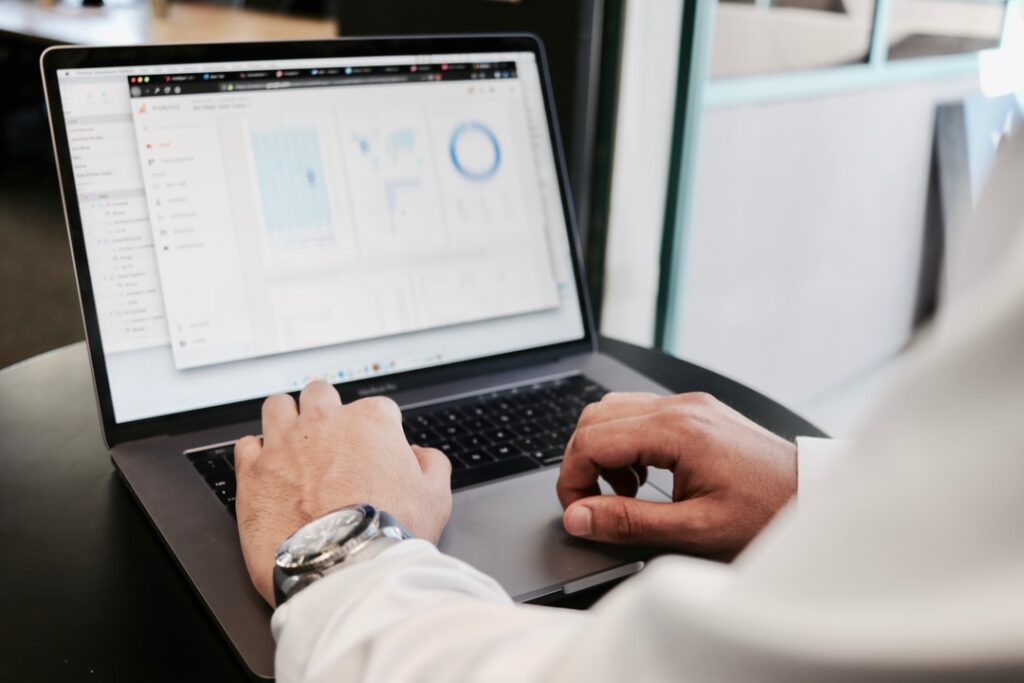
Organizations that are invested in their data standards are able to leverage data to make better decisions. With high-quality data, businesses are able to spend more time focusing on the outside and their customer base rather than the inner mechanisms as artificial intelligence runs on this better master data.
When many departments of an organization have constant access to the same high-quality data, the result is far superior. There is also better communication amongst members, making it easier to remain aligned in terms of priorities.
Also Read: Why Your Business Should Utilize Marketing Database Software
This makes for better messaging and branding to ensure the best results. With better data quality, companies are able to better assess customer interests and requirements. Taking the right data quality measures can be an absolute game-changer for companies to handle their different data sources.